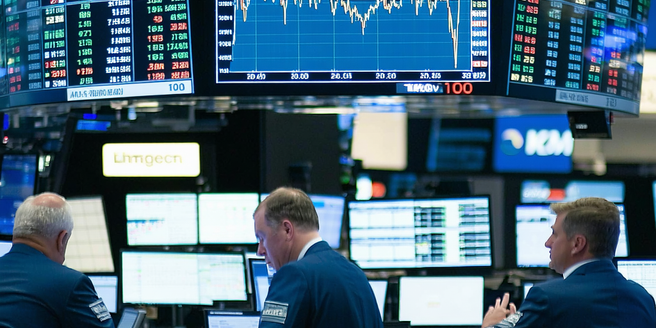
Introduction to Real-time Data Collection
Real-time data collection refers to the process of gathering and analyzing data as it is produced or acquired, enabling immediate action and decision-making. This level of immediacy is crucial for industries where timely response is key, such as finance, healthcare, and logistics. As businesses increasingly operate in fast-paced environments, the demand for real-time data analytics has soared. With the integration of IoT devices and advanced networking, the scope of data that can be collected in real-time has expanded significantly. This allows organizations to not only track operations as they happen but also to anticipate and mitigate potential issues. Moreover, investments in technologies that support real-time data flow are growing as companies recognize the value of agile and responsive business strategies.
Benefits of Real-time Data in Decision Making
Integrating real-time data into decision-making processes enhances organizational efficiency and agility. Real-time data provides up-to-the-minute insights, which are crucial in quickly evolving markets. This immediacy improves the accuracy of decisions, reducing the reliance on outdated or historical data that might not reflect current conditions. Companies can react promptly to unexpected changes, such as sudden shifts in consumer preferences or supply chain disruptions, allowing for swift strategy adjustments. Furthermore, real-time data supports predictive analytics, facilitating proactive rather than reactive decision-making. This capacity to make informed, immediate decisions not only optimizes resource allocation but also strengthens competitive advantage, enables personalized customer experiences, and improves overall business resilience in uncertain environments.
Challenges in Implementing Real-time Data Systems
Implementing real-time data systems presents several challenges, primarily centered around infrastructure and cost. Establishing an ecosystem that supports continuous data flow requires robust IT infrastructure, involving both hardware and software upgrades. Scalability becomes critical as data volumes grow, necessitating systems capable of managing large data streams without latency. The cost of implementation can be significant, encompassing not only technology investment but also ongoing maintenance and staff training. Furthermore, data accuracy and consistency pose risks, as flawed data can lead to misguided decisions. Security is another paramount concern, with real-time systems needing to be fortified against breaches to protect sensitive information. Lastly, integrating real-time data with existing legacy systems often proves complex, requiring significant time and resources to ensure compatibility and efficiency.
Popular Tools for Collecting Real-time Data
Numerous tools facilitate real-time data collection, each catering to different needs and industries. Apache Kafka is a popular open-source platform for building real-time data pipelines, enabling high-throughput data processing. For businesses requiring comprehensive analytics, Google Cloud’s BigQuery provides a serverless option that allows for the analysis of massive datasets with speed and accuracy. Amazon Kinesis offers real-time streaming data capabilities, supporting applications that require rapid, continuous data capture. For IoT applications, Microsoft Azure IoT Hub is ideal for connecting, monitoring, and managing billions of IoT assets. Tools like Tableau and Power BI are excellent for visualizing real-time data, turning raw numbers into actionable insights. The choice of tool depends on specific business requirements, data workloads, and existing infrastructure.
Best Practices for Effective Data Collection
Effective data collection involves strategies that ensure data quality and utility. Begin with a clear understanding of organizational data needs and objectives to tailor data collection processes accordingly. Implement standardized methods for data gathering to maintain consistency, and regularly audit data for accuracy. Use secure, scalable systems that can handle data growth while protecting against unauthorized access. Incorporate automation where possible to streamline data entry and reduce human error. Establish data governance policies to clarify roles, responsibilities, and procedures for data handling. Engage data stakeholders regularly to ensure systems evolve in line with business goals. Additionally, leverage feedback loops to adjust and refine data strategies continuously, ensuring that the insights gained are actionable and relevant.
Future Trends in Real-time Data Technology
The future of real-time data technology promises rapid advancements driven by AI, machine learning, and edge computing. AI will enhance data analytics capabilities, allowing for more sophisticated predictions and automated decision-making processes. As edge computing grows, data processing will increasingly occur at the source of generation, reducing latency and enhancing speed. This is particularly significant for IoT applications where data needs to be processed immediately rather than being sent to centralized locations. Furthermore, we can anticipate broader adoption of 5G technology, which will enable faster and more reliable data transmission, essential for real-time applications. Privacy and data protection will also evolve, with regulatory frameworks adapting to ensure that real-time data practices adhere to ethical standards and address emerging security challenges.