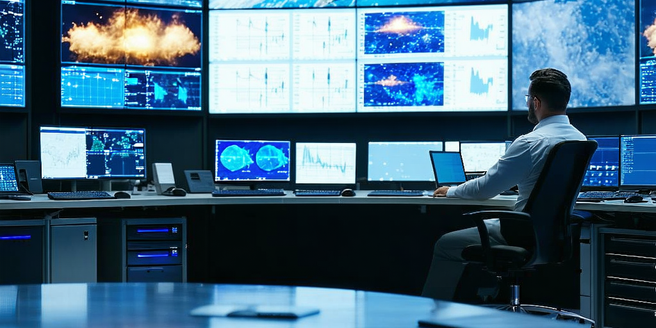
Understanding the Basics of Weather Prediction Models
Weather prediction models are essential tools used by meteorologists to forecast weather conditions. They are complex mathematical representations of the atmosphere that simulate weather dynamics over time. By using initial conditions from real-time data, these models predict future atmospheric states. Numerical weather prediction (NWP) forms the backbone of modern forecasting, harnessing the power of supercomputers to process vast datasets. However, it is crucial to understand model limitations, such as grid resolution and inherent approximations. By continually refining these models and incorporating new data, meteorologists enhance predictive accuracy. Additionally, ensemble forecasting, which runs multiple simulations to account for possible variations, is pivotal in improving reliability. Ultimately, understanding these models allows for better preparation and response to weather events.
The Role of Geographic Data in Localized Forecasting
Geographic data plays a pivotal role in enhancing the accuracy of localized weather forecasts. Topography influences weather patterns significantly, affecting variables like wind direction and precipitation. By integrating high-resolution geographic information into weather models, forecasters can better predict how local features impact weather conditions. For instance, mountainous regions can cause variations in temperature and precipitation that differ significantly from flatter areas. The inclusion of geographic data allows models to simulate these effects, leading to more accurate localized predictions. Furthermore, advancements in technology have made it possible to collect and analyze geographic data more efficiently than ever before. Additionally, understanding land-use patterns can provide insights into localized climate effects, further refining forecasts. This approach helps in tailoring warnings and advisories to specific communities.
Advancements in Remote Sensing for Storm Predictions
Remote sensing technology has revolutionized storm prediction by providing real-time atmospheric data. Satellites and radar systems offer critical insights into the structure and movement of storms, enabling more accurate predictions. For example, Doppler radar is utilized to detect wind patterns and storm intensity, which are critical for predicting severe weather events like tornadoes and hurricanes. Advancements in satellite imaging, such as higher spatial and temporal resolution, allow meteorologists to monitor storm development across vast regions. Additionally, the integration of machine learning algorithms with remote sensing data is increasingly being used to improve predictive models. These technologies also facilitate better tracking of storm paths and intensities, which is essential for timely warnings. By continually improving remote sensing capabilities, the accuracy and lead time of storm predictions can be significantly enhanced.
Incorporating Machine Learning into Local Weather Forecasts
The integration of machine learning into weather forecasting represents a significant advancement in predicting localized weather events. Machine learning models can analyze vast datasets and identify complex patterns that traditional forecasting methods might miss. By training algorithms on historical weather data, machine learning can enhance prediction accuracy by providing probabilistic forecasts. As technology evolves, these systems become more efficient and scalable. These models excel in short-term forecasting, where rapid changes are common. For instance, machine learning techniques can predict the likelihood of severe weather events, offering crucial lead times for preparation. Additionally, these models can adapt to new data, continuously refining their accuracy. The fusion of machine learning and traditional forecasting methods holds the potential to transform local weather predictions.
Challenges and Solutions in Predicting Microclimates
Predicting microclimates poses unique challenges due to their small scale and specific characteristics. Microclimates can be influenced by various factors such as topography, vegetation, and urban development, resulting in localized weather conditions different from the surrounding regions. To address these challenges, meteorologists use high-resolution models that incorporate detailed geographic and environmental data. These models simulate the intricate interactions between the atmosphere and surface features, allowing for precise microclimate predictions. Moreover, collaboration between different scientific disciplines plays a crucial role in enhancing these predictive models. Additionally, advancements in sensor technology enable the collection of localized meteorological data, further enhancing model accuracy. Despite the complexities, ongoing research and technology improvements continue to refine our ability to forecast microclimates effectively.