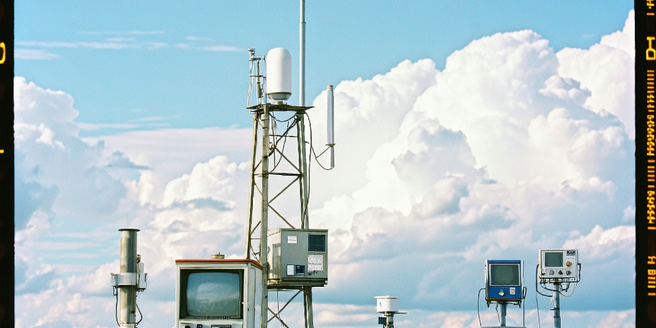
Understanding the Importance of Weather Data Analysis
Weather data analysis is crucial in predicting future climate patterns and informing disaster preparedness plans. By examining past weather records, researchers can identify trends, understand changes in climate, and assess the impact on various sectors such as agriculture, tourism, and public health. Accurate weather data enables policymakers to create better strategies for mitigating natural disasters and reducing economic losses. Moreover, this analysis allows scientists to study the correlation between human activities and climate change. In order to make informed decisions, access to reliable historical data is vital. It not only aids in academic research but also supports businesses in optimizing operations and minimizing weather-related disruptions. Thus, understanding weather data analysis extends beyond mere academic interest to a practical necessity for sustainable living.
Methods for Collecting Historical Weather Data
Collecting historical weather data involves an array of methods, each with its unique advantages. One common method is through traditional weather stations, which have been recording various weather parameters like temperature and precipitation for decades. Satellite data provides another vital source, offering extensive coverage and real-time monitoring capabilities. Additionally, historical documents, journals, and local folklore can offer valuable insights into weather patterns before instrumental records began. Remote sensing technology, such as radar, also plays a crucial role by tracking atmospheric phenomena and collecting data over vast geographical areas. Each of these methods contributes to a comprehensive dataset necessary for thorough analysis. Leveraging multiple data sources ensures a robust understanding of historical weather conditions, which is essential for making accurate predictions and informed decisions in the face of climate change.
Techniques for Cleaning and Organizing Data
Cleaning and organizing data is a critical step in weather data analysis, ensuring accuracy and reliability. Techniques for cleaning data include identifying and correcting errors, filling in missing data points, and removing outliers that could skew results. Organizing involves structuring data in a logical manner for easy access and analysis, which often includes sorting, classifying, and naming datasets consistently. Implementing standardized measurements and units is also crucial, as inconsistency can lead to misinterpretations. Data scientists employ software tools that automate cleaning processes, ensuring efficiency and consistency across large datasets. Good practices in data handling not only enhance the quality of the analysis but also improve reproducibility of results, fostering confidence in the findings. Well-organized data supports effective analysis, enabling stakeholders to derive meaningful insights relevant to weather trends.
Identifying Patterns in Weather Over Time
Identifying patterns in weather over time involves analyzing historical data to detect recurring trends and anomalies. Techniques such as time-series analysis, machine learning algorithms, and statistical models help in understanding these patterns. For instance, seasonal trends can provide insight into regular changes, while anomalies may indicate significant shifts in climate conditions. Recognizing consistent patterns aids in predicting future weather events, offering valuable foresight for farmers, urban planners, and policymakers. Long-term weather data allows scientists to study phenomena like El Niño and La Niña, understanding their impacts on global weather systems. Accurate identification and interpretation of these patterns contribute to climate modeling and risk assessment. Ultimately, identifying weather patterns supports proactive measures, helping mitigate adverse effects and guiding strategic planning across various sectors.
Case Studies: Weather Trends in Different Regions
Examining case studies of weather trends in different regions reveals the diverse impacts and adaptations to climate variability. For instance, regions prone to hurricanes, such as the Caribbean, have adapted infrastructure and emergency protocols to mitigate damage and enhance resilience. In contrast, arid regions like the Middle East face challenges in water scarcity and have developed innovative water conservation and management technologies. By studying these regional trends, we gain insights into how geographical and socio-economic factors influence weather adaptation strategies. Such case studies highlight the importance of localized research for developing targeted, effective climate response plans. Additionally, they offer lessons in resilience and adaptation that can be applied to other regions facing similar climatic challenges. Understanding these regional differences is crucial for comprehensive climate policy and international collaboration in addressing global weather phenomena.
Implications of Historical Weather Trends on Future Predictions
Assessing historical weather trends has profound implications for future predictions, serving as a cornerstone for climate models and risk assessments. By analyzing patterns and anomalies in past data, scientists can enhance the accuracy of predictive models, offering better forecasts for extreme weather events. This is pivotal for sectors like agriculture, where timely predictions aid in planning and resource allocation. Moreover, understanding historical trends informs adaptive strategies for infrastructure and public health. It also plays a crucial role in policy-making, providing evidence for climate change discussions and action plans. The increasing availability of comprehensive historical datasets, combined with advancements in computing, has improved forecasting capabilities. However, challenges remain due to factors like data gaps and the complex nature of climate systems. Continued research in this area is essential for refining future weather predictions and enabling a proactive approach to climate resilience.