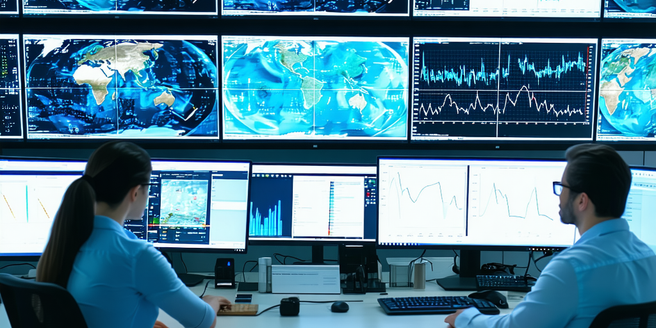
Introduction to Climate Forecasting Models
Climate forecasting models are crucial tools that provide insights into future climate changes. These models employ mathematical representations of the earth’s climate system, incorporating factors like ocean currents, wind patterns, and greenhouse gas concentrations. By simulating interactions among these components, scientists can predict climate variables such as temperature, precipitation, and storm patterns. Key to these models is the accuracy of input data and the assumptions made regarding future scenarios. Advances in computational capacity and data availability have dramatically improved model precision. Scientists continue to refine these models to account for uncertainties inherent in predicting complex climate systems. Understanding these forecasts is vital for policymakers, helping to guide mitigation and adaptation strategies against climate change impacts.
The Role of Probability in Climate Predictions
Probability plays a significant role in climate predictions, providing a framework to address the uncertainties inherent in forecasting complex systems. Statistical techniques are used to quantify the likelihood of various climate scenarios, drawing from historical data and model outputs. These probabilistic forecasts help convey the range of possible future climates, rather than a single deterministic outcome. They offer insights into extremes, such as the chance of severe weather events or shifts in average conditions, which are crucial for decision-making. Probability-based forecasts support strategies in risk management by articulating risks in adaptable terms. This probabilistic approach enhances the communication of scientific uncertainty, helping policymakers, stakeholders, and the public make informed decisions.
Time Series Analysis in Meteorology
Time series analysis is a fundamental tool in meteorology, helping to decipher patterns in climate data over time. This technique involves analyzing data points collected at successive points in time to identify trends, cycles, and anomalies. In climate science, this method is used to track changes in variables such as temperature, rainfall, and wind speed, offering insights into seasonal patterns and long-term climate shifts. Time series analysis aids in recognizing anomalies that could signal climate change phenomena, like increased frequency of heatwaves or shifts in monsoon patterns. It helps meteorologists, researchers, and policymakers understand both short-term weather phenomena and long-term climate trends, providing a basis for accurate climate predictions and informing adaptive strategies.
Using Regression Techniques for Climate Data
Regression techniques are vital in analyzing climate data, allowing researchers to explore relationships between dependent and independent variables. These statistical methods help quantify the impact of various factors, such as greenhouse gas concentrations or land use changes, on climate variables like temperature and precipitation. Linear regression can provide estimates of climate change trends, while more complex models like multiple regression can assess interactions among diverse variables. These techniques enhance understanding of how human activities influence climate systems, aiding in the development of reliable climate models. By analyzing past and present data trends, regression analysis contributes to improved accuracy in climate forecasting, supporting policy development focused on climate change mitigation and adaptation.
Machine Learning Applications in Weather Forecasting
Machine learning is transforming weather forecasting by enhancing the analysis of massive datasets, leading to more accurate and timely predictions. These techniques can automatically identify complex patterns and relationships in climate data that traditional models might miss. Algorithms like neural networks and decision trees are employed to model non-linear interactions within the climate systems, improving predictive accuracy. Machine learning models can assimilate vast amounts of data from satellites, ocean buoys, and weather stations, offering real-time updates. As data volumes grow, these models become more refined, providing critical information for weather alerts and climate predictions. The integration of machine learning in forecasting is set to advance the field significantly, paving the way for innovations in climate science.
Evaluating the Accuracy of Climate Projections
Evaluating the accuracy of climate projections is pivotal in building trust and effectiveness in climate science. This process involves comparing model outputs with observed data to identify strengths and weaknesses in forecasting capabilities. Validation techniques like back-testing and cross-validation are employed to assess model accuracy over different time frames. Discrepancies between projections and real-world outcomes are scrutinized to refine model algorithms and input data. By identifying the limits of current models, scientists can improve their design and predictive power. Regular evaluation ensures models remain relevant and credible, guiding policymakers in formulating response strategies to future climate scenarios. As climate systems evolve, continuous validation and refinement are essential to maintaining the reliability of climate projections.