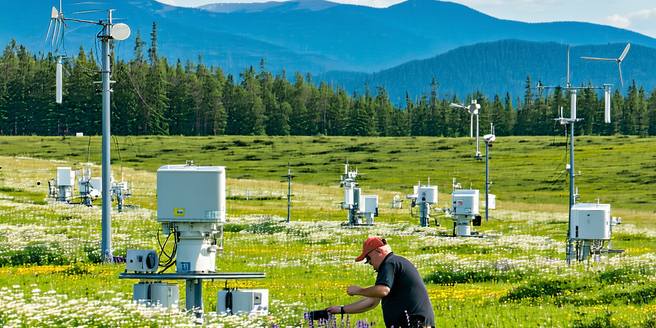
Introduction to Climate Data Sources
Climate data sources are diverse and include temperature, precipitation, and atmospheric pressure measurements from various instruments. Traditional sources encompass weather stations, satellites, and ocean buoys, all providing essential data for climate studies. Weather stations are distributed globally, offering long-term data series necessary for trend analysis. Satellites, meanwhile, deliver vast amounts of data covering the entire globe, essential for understanding planetary-scale processes. Ocean buoys provide critical insights into sea-surface temperatures and currents, both vital components of climate systems. Additionally, reanalysis datasets, which blend observations with model outputs, offer comprehensive climate records. These multiple data sources must be integrated meticulously to ensure accuracy in analysis and modeling. This integration often requires simultaneous verification against benchmark datasets, ensuring that the derived conclusions reflect reality.
Methods of Data Collection and Verification
Data collection methodologies have evolved to enhance the precision and accuracy necessary for reliable climate predictions. Instruments such as automated weather stations and satellite sensors collect vast datasets across temporal and spatial scales. Ground-truthing, where data collected from these instruments is compared against known reference points, is crucial for verification. Quality control processes further ensure data integrity by identifying and correcting errors in raw datasets. Additionally, rigorous calibration of instruments is conducted before and after deployment, ensuring consistent data collection. Peer-reviewed intercomparison studies offer additional levels of verification by assessing the accuracy and reliability of different data collection methods. Moreover, data assimilation techniques that integrate observational data into models improve predictive capabilities by adjusting model outputs closer to observed conditions. These methods collectively ensure the reliability of climate data for subsequent modeling efforts.
Statistical Techniques in Climate Modeling
Statistical techniques are vital in climate modeling, offering tools to decipher complex datasets and predict future conditions. Regression analysis, a foundational technique, helps identify relationships between climate variables, such as temperature and CO2 levels. Time series analysis is employed to detect trends and cycles, necessary for understanding phenomena like El Niño. Variance and anomaly detection are used to identify rare climate events or shifts. Machine learning algorithms, including neural networks and decision trees, are increasingly used to enhance predictive accuracy. These algorithms can process large datasets, identifying patterns that traditional methods may miss. Downscaling methods are employed to translate global model outputs to finer regional scales, crucial for local decision-making. These statistical tools provide the backbone for robust climate predictions, aiding policymakers in crafting informed climate strategies.
Machine Learning in Climate Predictions
Machine learning plays a transformative role in climate predictions, offering advanced methodologies to process complex datasets. With the ability to handle large-scale data efficiently, machine learning models, such as neural networks, can discern patterns that remain elusive to traditional methods. Techniques like deep learning have enabled advancements in predicting extreme weather events, such as cyclones and heatwaves, with improved accuracy. These models learn from historical data, refining their predictions as more data becomes available. Transfer learning, another innovative technique, allows models trained on one type of data to be adapted for different scenarios, increasing the flexibility and applicability of predictive models. Reinforcement learning optimizes these models by rewarding accurate predictions and adjusting parameters accordingly. As machine learning technologies evolve, they will continue to be integral in enhancing the precision of climate predictions.
Challenges and Limitations of Current Models
Current climate models, while powerful, face several challenges and limitations. One major issue is the inherent uncertainty in predicting complex climate systems, compounded by incomplete understanding of feedback mechanisms. Resolution limitations mean that local-scale phenomena, such as thunderstorms, are often not captured accurately. Additionally, differences in model configurations and parameterizations can lead to variance in model outputs. Computational constraints hinder the ability to run high-resolution simulations over long timescales, impacting long-term forecasting capabilities. Intermodel discrepancies also pose challenges, with different models sometimes producing divergent outcomes for similar scenarios. Furthermore, integrating socio-economic factors into climate models remains a complex task, necessitating interdisciplinary approaches. Addressing these limitations requires advancements in computing power, improved data assimilation techniques, and enhanced understanding of climate processes to ensure models are both accurate and reliable.
Future Directions in Climate Data Analysis
The future of climate data analysis holds exciting prospects driven by advancements in technology and methodology. Emerging trends include the integration of big data analytics, leveraging vast datasets to uncover insights previously obscured. Enhanced satellite technology promises finer spatial and temporal resolution data, crucial for improving model accuracy. Interdisciplinary approaches, combining climate science with fields like data science and computational modeling, hold promise for developing comprehensive climate solutions. There is also a push towards democratizing data, ensuring that open-access datasets are available to researchers worldwide, fostering collaboration and innovation. Machine learning and artificial intelligence will likely play an increasingly pivotal role in analyzing complex datasets, enabling faster and more accurate predictions. As these technologies evolve, they will pave the way for more resilient, adaptive strategies to mitigate and adapt to climate change challenges.