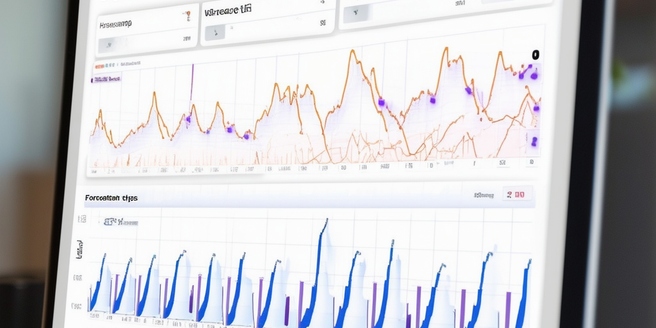
Introduction to Real-time Forecasting
Real-time forecasting refers to the use of advanced data analysis tools to predict future trends and outcomes almost instantaneously. This process involves the continuous collection and analysis of data as it’s generated, allowing businesses and organizations to make immediate, data-driven decisions. Traditional forecasting methods often rely on historical data, which can lead to a lag in response times. However, real-time forecasting leverages new technology tools, such as machine learning and artificial intelligence, to offer a more dynamic and responsive approach. By utilizing real-time data inputs, organizations can anticipate changes and trends much sooner, which is crucial in today’s fast-paced market environments. This proactive stance not only aids in better decision-making but also provides companies with a competitive edge over those dependent on traditional, slower methods of data analysis.
Benefits of Real-time Forecasting in Business
Implementing real-time forecasting techniques allows businesses to navigate today’s rapidly changing environments with greater agility and confidence. One of the primary advantages is improved decision-making capabilities. By integrating continuous data analysis into their operations, companies can respond to market demands in real time, minimizing risks and capitalizing on opportunities as they arise. This ability to act swiftly can lead to increased efficiency, cost savings, and competitive advantages. Furthermore, real-time forecasting supports better inventory management and demand planning, as businesses can quickly adjust their strategies based on current market conditions. Additionally, it enhances customer satisfaction by predicting needs and optimizing resources to meet demands promptly. Ultimately, real-time forecasting is an invaluable tool for businesses aiming to thrive in the modern, data-driven economy.
Key Technologies Enabling Real-time Predictions
The realm of real-time forecasting relies heavily on several key technologies that enable rapid data processing and actionable insights. Among them, machine learning algorithms are pivotal, as they can process vast amounts of data to identify patterns and trends faster than traditional methods. Moreover, cloud computing plays a significant role by providing the necessary computational power and storage capabilities to handle data in real time. The use of big data analytics allows for the examination of large datasets to glean valuable insights. Additionally, the integration of the internet of things (IoT) devices offers continuous data streams that enhance the accuracy of forecasts. Finally, artificial intelligence contributes through advanced predictive models that learn over time. Together, these technologies form the backbone of real-time forecasting, enabling swift and accurate predictions that organizations rely upon to stay ahead.
Case Studies: Success Stories in Real-time Forecasting
Real-time forecasting has revolutionized many industries, leading to remarkable success stories. Consider the retail sector, where a major online retailer leveraged real-time data analytics to optimize its supply chain, reducing delivery times by 30%. This adaptation not only improved customer satisfaction but also led to a significant increase in repeat business. In the financial industry, a large bank applied real-time forecasting to its risk management protocols, identifying potential fraud activities as they arose, thereby safeguarding customer assets and enhancing trust. In manufacturing, a company utilized real-time predictive maintenance techniques, resulting in a 25% reduction in equipment downtime. These examples illustrate how diverse sectors can harness the power of real-time forecasting, not only to solve immediate challenges but also to create lasting competitive advantages and drive innovation.
Future Trends in Real-time Forecasting Innovation
As businesses continue to seek out competitive advantages, the landscape of real-time forecasting is poised for significant advancements. One major trend is the integration of augmented and virtual reality in data visualization, allowing for more intuitive and immersive interaction with data. Additionally, the advancement of edge computing promises to further decrease latency and enhance decision-making at the source of data collection. Blockchain technology also holds potential to offer enhanced security and transparency in data transactions crucial for reliable real-time forecasting. Moreover, advancements in AI will lead to even more sophisticated predictive analytics models, increasing the accuracy and reliability of forecasts. Finally, as IoT devices become more prevalent, the volume of real-time data available for analysis will increase exponentially, offering richer insights. These innovations are set to redefine how businesses utilize real-time forecasting to stay competitive.