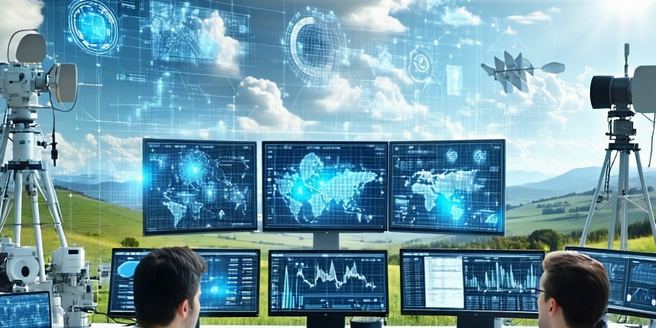
Understanding Climate Models
Climate models are complex systems-driven tools that simulate the Earth’s climate over various temporal scales. These models are designed to represent the interactions between the atmosphere, oceans, land surface, and ice. They use mathematical equations based on fundamental laws of physics, climate science, and data observations. By simulating the climate system, scientists can predict long-term climate patterns, such as temperature and precipitation changes, on a global scale. These models serve as crucial tools in assessing the impact of human activities on climate and aiding policymakers in developing strategies to mitigate climate change. Moreover, they help in understanding natural climate variability and extreme weather events. Despite their capability in predicting general trends, climate models face limitations such as spatial resolution and uncertainties in future projections due to the complexity of climate systems.
Delving into Weather Models
Weather models, differing from climate models, are used to simulate and predict short-term weather conditions, usually focused on periods ranging from hours to days. They operate on finer spatial and temporal scales compared to climate models. By integrating real-time atmospheric data, such as temperature, humidity, wind speed, and pressure, these models deliver forecasts used by meteorologists to predict local weather events. Numerical weather prediction (NWP), a key methodology, involves solving mathematical equations that describe atmospheric flows. The models require immense computational power and depend heavily on data accuracy for precise forecasts. While they are continually refined to improve prediction accuracy, their outputs can still be affected by unpredictable short-term variables. Advances in technology, satellite observations, and data assimilation techniques continue to enhance model precision.
Key Differences Between Climate and Weather Models
Climate and weather models, while similar in using physical laws and mathematical equations, primarily differentiate based on their application focus and time scales. Climate models predict long-term patterns and trends across decades to millennia, capturing the intrinsic variability within Earth’s climate system and anthropogenic impacts, like greenhouse gas emissions. In contrast, weather models are intended for short-term forecasts, dealing with atmospheric conditions over days to weeks. They’re adept at handling local forecasts and specific meteorological events. The differences also extend to the spatial resolution: climate models offer coarse resolution suitable for capturing large-scale phenomena, whereas weather models provide detailed resolutions needed for precise event forecasting. Understanding these key distinctions helps when interpreting model outputs and their respective purposes in scientific research and operational forecasts.
Applications and Limitations
Both climate and weather models serve vital roles in scientific research and practical applications. Climate models, essential for understanding long-term global changes, inform international climate agreements and guide resource management strategies. Weather models support day-to-day decision-making in aviation, agriculture, emergency management, and various industries reliant on weather forecasts. Despite their strengths, both model types face limitations. Climate models struggle with accurately representing small-scale processes and their interactions. Weather models, although becoming increasingly precise, often have difficulties with sudden weather changes, necessitating constant updates and complex data assimilation. Furthermore, both models entail challenges regarding computational power and data availability. Continuous research aims to bridge these limitations through advanced technologies, improved algorithms, and collaborative international efforts.
Future Advancements in Modeling Technology
Innovations in modeling technology promise enhanced accuracy and reliability in both climate and weather predictions. Emerging advancements include increased computational power, allowing for higher resolution models and more detailed simulations. Integration of artificial intelligence and machine learning techniques is reshaping data assimilation processes, yielding better model initialization and outcomes. Satellites and remote sensing technologies continue to provide rich datasets, which can be utilized for improving model inputs and enhancing the capture of climate and atmospheric dynamics. Moreover, international collaborations are fostering the development of unified modeling frameworks, efficiently combining climate and weather forecasting systems. These advancements aim to reduce uncertainties in predictions, potentially transforming our understanding of the Earth’s systems. However, the complexity of climate feedback mechanisms and unpredictable natural variabilities remain challenges for future innovations.