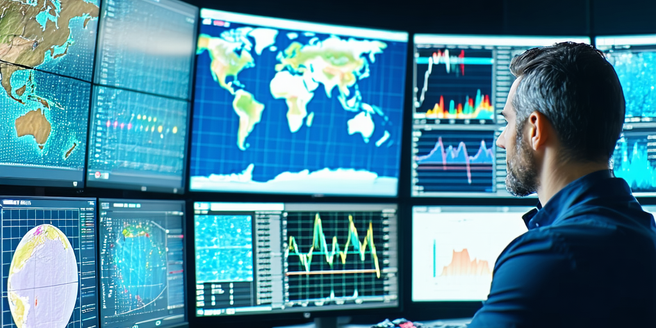
The Basics of Climate Models
Climate models are sophisticated tools that use mathematical equations to simulate the interactions of the atmosphere, oceans, land surface, and ice. By incorporating various physical, chemical, and biological properties, these models aim to represent the Earth’s climate system. Scientists use climate models to understand past climate conditions and predict future changes. The accuracy of these models depends on the precision of the data input and the way different climate components are represented. Through increasing computing power, these models have become more detailed and are essential for studying climate variability such as El Niño events. The models can run simulations under different scenarios, accounting for anthropogenic factors like greenhouse gas emissions. This helps in assessing potential future impacts, guiding policymakers in decision-making processes.
Types of Climate Models Explained
Climate models can be broadly classified into different types based on complexity and scope. The simplest ones are Energy Balance Models (EBMs) which focus on the Earth’s energy inputs and outputs. General Circulation Models (GCMs), on the other hand, provide detailed simulations by dividing the Earth system into 3D grids, allowing for more accurate representation of the atmospheric and oceanic processes. Regional Climate Models (RCMs) add another layer of detail by focusing on specific regions, providing insights about local climate impacts. Earth System Models (ESMs) include additional components like the carbon cycle and biosphere, offering a more comprehensive picture. Each model type has its use case, emphasizing a balance between computational efficiency and detailed representation of climate processes.
Components and Variables in Climate Models
Climate models integrate various components of the Earth system, such as the atmosphere, oceans, land surface, and ice to simulate the climate. Each component has associated variables that are crucial for accurate modeling. For instance, the atmospheric component considers temperature, humidity, wind patterns, and atmospheric pressure. The oceanic component takes into account sea surface temperature, salinity, and ocean currents. Land surface processes include soil moisture, vegetation cover, and albedo. Ice components model the dynamics of glaciers and sea ice extent. The interaction between these components must be accurately represented to reflect climate feedback mechanisms. This complexity requires a deep understanding of not only individual component processes but also how they interconnect, influencing the overall climate system.
How Climate Models Predict Future Change
Climate models predict future changes by running simulations based on different scenarios of greenhouse gas emissions and other anthropogenic factors. These scenarios, known as Representative Concentration Pathways (RCPs), offer projections ranging from low to high emissions futures. To enhance their accuracy, models are continually updated with the latest climate data and scientific findings. The models process past and present climate data, providing a baseline for future climate projections, including temperature fluctuations, precipitation changes, and extreme weather events. Advanced statistical techniques are employed to evaluate uncertainty and sensitivity, ensuring more robust predictions. Through these simulations, climate models help in forecasting sea-level rise, shifts in agricultural zones, and biodiversity impacts. The insights gained are vital for adaptation strategies, promoting resilient infrastructure, and informing policymakers on environmental and economic planning.
Challenges and Limitations of Climate Modeling
Despite their sophistication, climate models face several challenges and limitations. One significant hurdle is the precise representation of complex processes such as cloud formation and ocean-atmosphere interactions. These are inherently chaotic, leading to varying degrees of uncertainty in predictions. Computational constraints also limit the resolution at which these models operate. Another issue is the quality and availability of input data, which can affect model accuracy. Regional models might not fully capture local climatic events due to insufficient granularity. Furthermore, models are based on assumptions that may not always account for unexpected natural events or socio-economic changes impacting emission pathways. Continued research and advancements in computing technology are essential to improve the reliability and accuracy of climate models.